Tachyum prodigy native AI supports TensorFlow and PyTorch
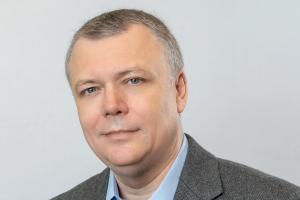
Radoslav Danilak of Tachyum
Tachyum Inc. today announced that it has further expanded the capabilities of its Prodigy Universal Processor through support for TensorFlow and PyTorch environments, enabling a faster, less expensive and more dynamic solution for the most challenging artificial intelligence/machine learning workloads.
Analysts predict that AI revenue will surpass $300 billion (€354 billion) by 2024 with a compound annual growth rate (CAGR) of up to 42% through 2027. AI is being heavily invested in by technology giants looking to make the technology more accessible for enterprise use-cases. They include self-driving vehicles to more sophisticated and control-intensive disciplines like Spiking Neural Nets, Explainable AI, Symbolic AI and Bio AI. When deployed into AI environments, Prodigy is able to simplify software processes, accelerate performance, save energy and better incorporate rich data sets to allow for faster innovation.
Proprietary programming environments like CUDA are inherently hard to learn and use. With open source solutions like TensorFlow and PyTorch, there are a hundred times more programmers that can leverage the frameworks to code for large-scale ML applications on Prodigy. By including support for deep learning environments that are easier to learn, build and train diversified neural networks, Tachyum is able to overcome and move beyond the limitations facing those working exclusively with NVIDIA’s CUDA or with OpenCL.
In much the same way that external floating-point coprocessors and vector coprocessor chips have been internalised into the CPU, Tachyum is making external matrix coprocessors for AI an integral part of the CPU. By having integrated matrix operations as part of Prodigy, Tachyum is able to provide high-precision neural network acceleration of up to 10 times faster than other solutions. Tachyum’s support of 16-bit floating point and lower precision data types improves performance and saves energy in applications, such as video processing. Faster than the NVIDIA A100, Prodigy uses compressed data types to allow larger models to fit in memory. Instead of 20GB shared coherent memory, Tachyum allows 8TB per chip and 64TB per node.
Idle Prodigy-powered universal servers in hyperscale data centres, during off-peak hours, will deliver 10x more AI Neural Network training/inference resources than currently available, CAPEX free (i.e. at low cost, since the Prodigy-powered universal computing servers are already bought & paid for). Tachyum’s Prodigy enables edge computing and IOT products, which will have an onboard high-performance AI inference optimised to exploit Prodigy-based AI training from either the cloud or the home office.
“Business and trade publications are predicting just how important AI will become in the marketplace, with estimates of more than 50% of GDP growth coming from it,” said Dr. Radoslav Danilak, Tachyum founder and CEO. “What that means is that the less than 1% of data processed by AI today will grow to as much as 40% and the 3% of the planets power used by datacentres will grow to 10% in 2025. There is an immediate need for a solution that offers low power, fast processing and easy of use and implementation. By incorporating open source frameworks like TensorFlow and PyTorch, we are able to accelerate AI and ML into the world with human-scale computing coming in 2 to 3 years.”
Tachyum’s Prodigy can run HPC applications, convolution AI, explainable AI, general AI, bio AI and spiking neural networks, as well as normal data centre workloads on a single homogeneous processor platform with its simple programming model. Using CPU, GPU, TPU and other accelerators in lieu of Prodigy for these different types of workloads is inefficient.
A heterogeneous processing fabric, with unique hardware dedicated to each type of workload (e.g. data centre, AI, HPC), results in underutilisation of hardware resources, and a more challenging programming environment. Prodigy’s ability to seamlessly switch among these various workloads dramatically changes the competitive landscape and the economics of data centres.
Prodigy significantly improves computational performance, energy consumption, hardware (server) utilisation and space requirements compared to existing chips provisioned in hyperscale data centres today. It will also allow Edge developers for IoT to exploit its low power and high performance, along with its simple programming model to deliver AI to the edge.
Prodigy is truly a universal processor. In addition to native Prodigy code, it also runs legacy x86, ARM and RISC-V binaries. And, with a single, highly efficient processor architecture, Prodigy delivers performance across data centre, AI, and HPC workloads. Prodigy, the company’s flagship Universal Processor, will enter volume production in 2021. In April, the Prodigy chip successfully proved its viability with a complete chip layout exceeding speed targets. In August, the processor is able to correctly execute short programs, with results automatically verified against the software model, while exceeding the target clock speeds. The next step is to get a manufactured wholly functional FPGA prototype of the chip later this year, which is the last milestone before tape-out.
Prodigy outperforms the fastest Xeon processors at 10x lower power on data centre workloads, as well as outperforming NVIDIA’s fastest GPU on HPC, AI training and inference. A mere 125 HPC Prodigy racks can deliver 32 tensor EXAFLOPS. Prodigy’s 3X lower cost per MIPS and 10X lower core power translates to a 4X lower data centre Total Cost of Ownership (TCO), enables billions of dollars of savings for hyperscalers such as Google, Facebook, Amazon, Alibaba, and others. Since Prodigy is the world’s only processor that can switch between data centre, AI and HPC workloads, unused servers can be used as CAPEX-free AI or HPC cloud, because the servers have already been amortised.
Comment on this article below or via Twitter @IoTGN