Driving smarter: Solving the challenges of autonomous vehicles with AI
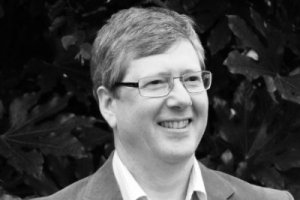
John Redford, VP Architecture, FiveAI
There is no longer any doubt that autonomous vehicles (AVs) will soon be a reality on our roads. However, there are multiple challenges which must first be overcome. Initial AVs will be restricted to operating on certain “known” roads, plus these early vehicles won’t be truly autonomous and will require human control and oversight at times.
Early deployments will probably be restricted to highway environments initially, and AVs will need the ability to pass the driving task back to a human when scenarios on the road get too complex.
Despite acres of newsprint and opinion, autonomous driving in the urban environment remains a largely unsolved challenge. Solving this puzzle will deliver massive benefits in terms of increased safety and driver leisure time, and reductions in pollution, congestion and cost, says John Redford, VP Architecture, FiveAI.
When AVs can safely deliver end-to-end journeys anywhere (defined by the SAE as Level 5 autonomy), with zero occupancy where necessary, there will be a dramatic shift away from the car ownership levels we see today towards mobility as a service (think about an Uber-like service, but the car will drive itself).
To get to this point we must surpass many implementation challenges. This is highly complex technology which must be built using commercially feasible hardware. We will also need to test and validate to ensure the technology is safe.
Major scientific challenges also remain, and these can be split roughly under two headings:
- Perception, i.e. what is happening right now in a given environment
- Intention Modelling, i.e. what will happen in the future of this environment
There is a natural distinction between these two problem spaces, but solving both of these challenges will require novel artificial intelligence (AI) applications. There is a single, determinable truth that describes what is happening in the instant and what has happened until that instant in a given scene.
Some factors are knowable: the instantaneous position of objects and actors relative to the ego-vehicle, their velocity and state, their type and pose, etc. Gaining this information requires a system to know precisely what is happening in a given scene and is delivered by both sensor and software performance, but also its position of observation, i.e. occlusions to the scene, lighting and other environmental hazards all affect the ability of an AV to observe.
So superior design of a perception system will enable more accurate knowledge of precisely what is happening in a scene, but this will be limited by the observation perspective (what we call “field of vision” in a human).
Computer vision is used to interpret this data, a field which has advanced in leaps and bounds recently. 2012 saw a significant breakthrough when convolutional neural networks (CNNs) were demonstrated to vastly improve image classification tasks over previous state-of-the-art technologies. Those techniques have advanced further since, and software can now outperform humans in most visual tasks required for driving.
This better-than-human perception ability will help deliver autonomous vehicles that are far less likely to suffer collisions than human drivers. But for autonomous vehicles to gain public acceptance they will have to drive in a way that other (human) road users expect, and they will have to make progress in busy traffic – driving assertively where necessary. This means AVs need the ability to anticipate what is likely to happen next in a scene. This remains an unsolved – and critical – challenge.
The future is intrinsically uncertain. Previously hidden objects or actors can enter the scene, and dynamic objects in the scene are also unpredictable. Autonomous vehicles must continually evaluate the possibilities of scene evolution to predict hazards that could arise, at almost any possibility level.
In real-time, AVs need to infer beliefs of the intentions of each actor in a scene and use learnt motion behaviours (relative to road topologies) to run probabilistic real-time modelling to determine possible actor paths consistent with those policies.
Intention modelling will rely on AI techniques such as counterfactual reasoning to interrogate “perceived” against “predicted” motion, thus ensuring AVs behave like other human road users, avoids collisions and find cooperative-competitive behaviours to avoid motion freezes.
These areas of emerging science have been gathering momentum in academic circles over the last 10 years. At FiveAI, we’re taking the best-in-class of this research and implementing it as automotive grade software, and developing the test and validation strategies to prove its safety. In doing so, autonomous vehicles will move from science fiction into reality, with all the associated societal benefits.
The author of this blog is John Redford, VP Architecture, FiveAI
Comment on this article below or via Twitter @IoTGN