Bright Box launches self-driving car with neural network that learns extreme driving from computer games
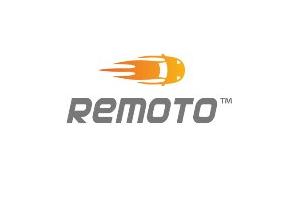
A European vendor of connected-vehicle applications like Nissan Smart Car app in Middle East, and KIA Remoto app in Russia, announced the launch of a self-driving car solution with a neural network trained through computer games as well as real-life examples.
Remoto Pilot, a new self-driving car solution, features safe, reliable road/lane following as well as real-time detection and avoidance of various obstacles such as cars and pedestrians. The key enabling technology for high efficiency autonomous driving capability of Remoto Pilot is the use of stereo vision in combination with advanced computer vision algorithms based on neural networks. Combined with the use of Global Navigation Satellite System (GNSS) and high-definition (HD) maps, this technology enables the possibility of fully autonomous car operation.
Currently, Bright Box’s main product is Remoto, a turnkey Connected Car platform that helps car owners to manage their cars remotely via smartphones (starting the engine, opening/closing doors, car tracking), provides large amounts of data to automotive and insurance companies, including information about cases of car malfunction, mileage, driver’s behavior, road accidents, etc. Company’s customers include such car makers as Nissan, Toyota, KIA, Infiniti.
Today the company announced launching autonomous driving solution designed to be a retrofit kit for existing cars.
Advanced computer-vision technologies, such as convolutional neural networks (СNN) with deep learning, in combination with stereo vision, can greatly enhance capabilities of self-driving cars. This technology allows for safe, reliable road/lane following, real-time detection and avoidance of various obstacles, such as cars, pedestrians etc.
The use of stereo vision (a pair of video cameras mounted on a car) allows for computation of distances to various objects in the field of view of the cameras. Thus, real-time assessment of the road situation by the car’s onboard computer becomes possible.
The training of a neural network involves the use of pre-computed representative sets of road situation video samples, referred to as training datasets.
One really amazing modern day technology that comes in handy for the neural network training is 3D computer graphics used in computer games. Such games, as GTA-V, with a large part of their gameplay involving driving on city streets, have a large number of extremely realistic city street views, as viewed from inside the cabins of driving cars, which makes them a very valuable source of high fidelity imagery that can be used to generate training datasets.
Two examples of training datasets, generated from GTA-V computer game, are shown below.
The original source images (shown on the left) are used to tell the neural network what various objects in a road scene look like, while the annotated images (shown on the right) are used to tell the neural network what kinds of objects are where.
Real-life training datasets, recorded from onboard cameras installed on real cars driving on real roads, are also used for neural network training. An example of a pair of images from a real-life training dataset is shown below.
Real-life training datasets, together with synthetic datasets generated from computer games, constitute a very efficient set of training samples used to train neural networks to analyse real-life road situations with high efficiency, thus providing the basis for safe and reliable autonomous driving on city streets.
One great advantage of this approach is the flexibility inherent in the neural network algorithms. While the neural network is trained on a limited number of samples, representing a limited number of road situations, it can correctly analyse a much larger number of road situations that differ in many ways from the training samples.
Neural networks can also be trained to measure distances from a stereo pair of cameras to various objects by using training datasets that include a number of pairs of images recorded from stereo cameras installed on real cars driving on real roads.
One common type of a sensor used in self-driving cars is a Lidar (a laser scanner that measures distances to surrounding objects), usually installed on a car’s rooftop.
The use of stereo cameras is an alternative to the use of Lidars. Both stereo cameras and Lidars measure distances to objects and can be used to generate depth maps that can be used for car trajectory planning.
Making a heavy emphasis on the use of advanced computer vision techniques, the company aims to develop technology that will eliminate the need for the use of Lidars.
Combined with the use of Global Navigation Satellite System (GNSS) and high-definition (HD) maps, this advanced computer vision technology enables the possibility of fully autonomous car operation.
Solution for OEMs
“We’ve got successful experience in connected-car solutions for remote car control and more than 250,000 cars connected. Today we work with a broad customer base among OEMs around the world (Europe, Middle East, Asia). The company is already negotiating solution supplies with OEMs and their partners, and hopes to increase the number of the company’s partners in the future. We also offer subscription based business model without significant R&D costs for OEMs”, said Bright Box’s CTO, Alexander Dimchenko.
Comment on this article below or via Twitter @IoTGN